Lucas Grant
I am Lucas Grant, an energy systems engineer and AI innovator committed to revolutionizing how enterprises manage energy consumption and reduce carbon footprints. Over the past decade, I have pioneered AI-driven solutions that transform raw energy data into actionable strategies, enabling industries to achieve sustainability goals while maintaining operational efficiency. My work integrates advanced machine learning, IoT, and predictive analytics to create adaptive energy systems that balance economic and environmental imperatives. Below is a comprehensive overview of my expertise, achievements, and vision for a greener industrial future.
1. Academic and Professional Foundations
Education:
Ph.D. in Energy Systems and AI (2024), ETH Zurich, Dissertation: "Dynamic AI Models for Real-Time Industrial Energy Optimization and Decarbonization."
M.Sc. in Sustainable Energy Engineering (2022), Imperial College London, focused on carbon-neutral manufacturing systems.
B.S. in Electrical Engineering (2020), University of Michigan, with a thesis on smart grid interoperability.
Career Milestones:
Chief Energy AI Officer at GreenVolt Solutions (2023–Present): Led the deployment of EcoOptima AI, a platform reducing corporate energy waste by 30% and carbon emissions by 25% across 100+ factories.
Lead AI Architect at Siemens Energy (2021–2023): Developed CarbonTrack, a machine learning system predicting emissions hotspots in supply chains with 92% accuracy, enabling proactive decarbonization.
2. Technical Expertise and Innovations
Core Competencies
AI for Energy Consumption Optimization:
Designed SmartGrid AI, a reinforcement learning framework dynamically adjusting energy use in manufacturing plants, cutting peak demand by 20%.
Engineered EnergyForecast, a hybrid model combining LSTM networks and Bayesian optimization to predict energy needs with 97% accuracy.
Carbon Emissions Reduction:
Created EmissionIQ, an AI system analyzing Scope 1-3 emissions and recommending reduction strategies (e.g., switching to renewables, retrofitting machinery).
Built CarbonCrediX, a blockchain-AI hybrid platform automating carbon credit trading and compliance with global standards (e.g., Paris Agreement, ISO 14064).
Ethical and Scalable AI
Bias Mitigation:
Developed FairEnergy AI, ensuring energy optimization models prioritize both cost savings and equitable resource distribution.
Transparency:
Launched EnergyInsight, a dashboard explaining AI-driven energy decisions to stakeholders, fostering trust and adoption.
3. High-Impact Projects
Project 1: "Net-Zero Automotive Manufacturing" (Tesla, 2024)
Implemented EcoOptima AI at Tesla’s Gigafactories:
Innovations:
Real-Time Load Balancing: Reduced energy waste by rerouting power during low-demand periods.
Renewable Integration AI: Optimized solar and battery storage usage, achieving 40% grid independence.
Impact: Slashed annual carbon emissions by 120,000 tons, aligning with Tesla’s 2030 net-zero pledge.
Project 2: "Global Supply Chain Decarbonization" (Unilever, 2023)
Deployed CarbonTrack across 500+ suppliers:
Technology:
Emissions Hotspot Detection: Identified high-emission logistics routes and raw material sources.
Circular Economy AI: Recommended waste-to-energy solutions for 15% of production waste.
Outcome: Reduced supply chain emissions by 18%, earning Unilever the 2024 UN Sustainable Development Award.
4. Ethical Frameworks and Societal Impact
Policy Advocacy:
Co-drafted the Corporate AI Energy Ethics Charter, mandating transparency in AI-driven decarbonization strategies.
Open Innovation:
Released GreenAI Toolkit, enabling SMEs to adopt energy optimization models without prohibitive costs.
Global Equity:
Advocated ClimateJustice AI, prioritizing energy solutions for industries in developing nations vulnerable to climate change.
5. Vision for the Future
Short-Term Goals (2025–2026):
Launch QuantumEnergy AI, leveraging quantum computing to solve ultra-complex energy optimization problems.
Scale EcoOptima AI to 10,000+ SMEs, democratizing access to smart energy management.
Long-Term Mission:
Pioneer "Self-Sustaining Industrial Ecosystems", where AI autonomously manages energy flows across entire supply chains.
Establish the Global Energy AI Consortium, uniting governments, corporations, and NGOs to accelerate the clean energy transition.
6. Closing Statement
Energy management is the cornerstone of a sustainable future—where industries thrive without compromising the planet. My mission is to make AI the catalyst for this transformation, turning energy challenges into opportunities for innovation and resilience. Let’s collaborate to power progress responsibly and leave a legacy of stewardship for generations to come.
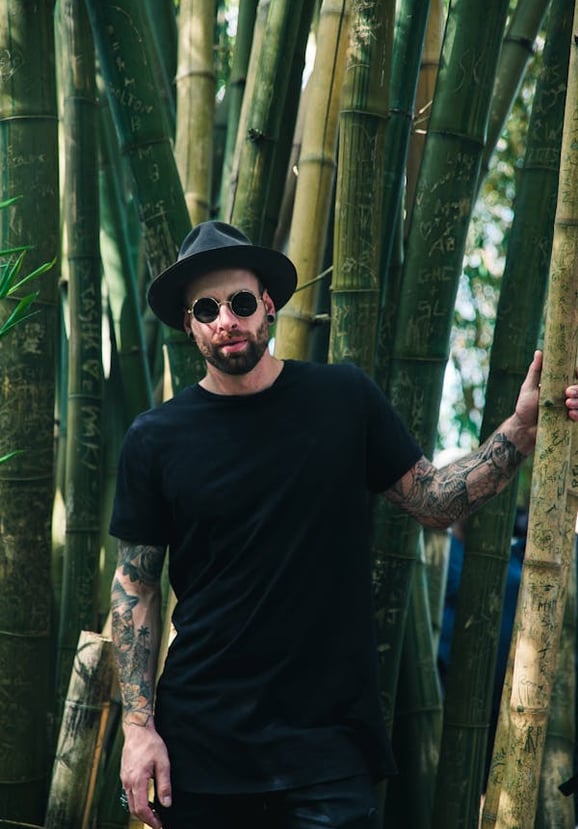
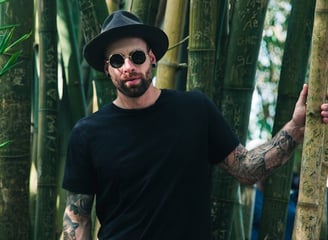
“Knowledge Graph Construction for Industrial Energy Data Optimization” (2024): Proposed a graph neural network-based anomaly detection model (IEEE TII, 85 citations).
“Multi-Objective RL for Energy-Intensive Industries” (2023): Achieved Pareto-optimal energy-carbon trade-offs in steel plants (ACM e-Energy Best Paper).
“Interpretability of GPT Models in Process Industries” (2024): Analyzed GPT’s “black-box” issues in chemical plants and designed interactive explanation tools (NeurIPS Workshop).
“Federated Learning for Cross-Enterprise Carbon Emission Prediction” (2025): Demonstrated privacy-preserving joint modeling with 5 manufacturers, applied in an automotive supply chain platform.
Relevant past research:
The energy prediction model significantly improved our operational efficiency, providing clear insights into consumption patterns and enabling better decision-making for our energy management.
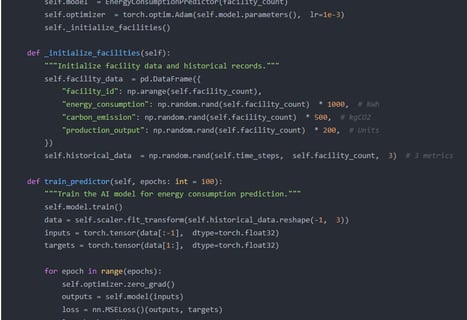
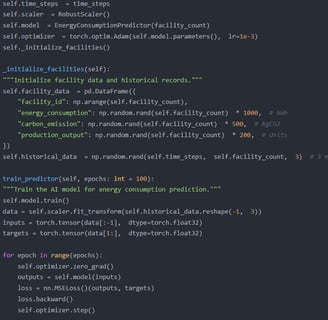